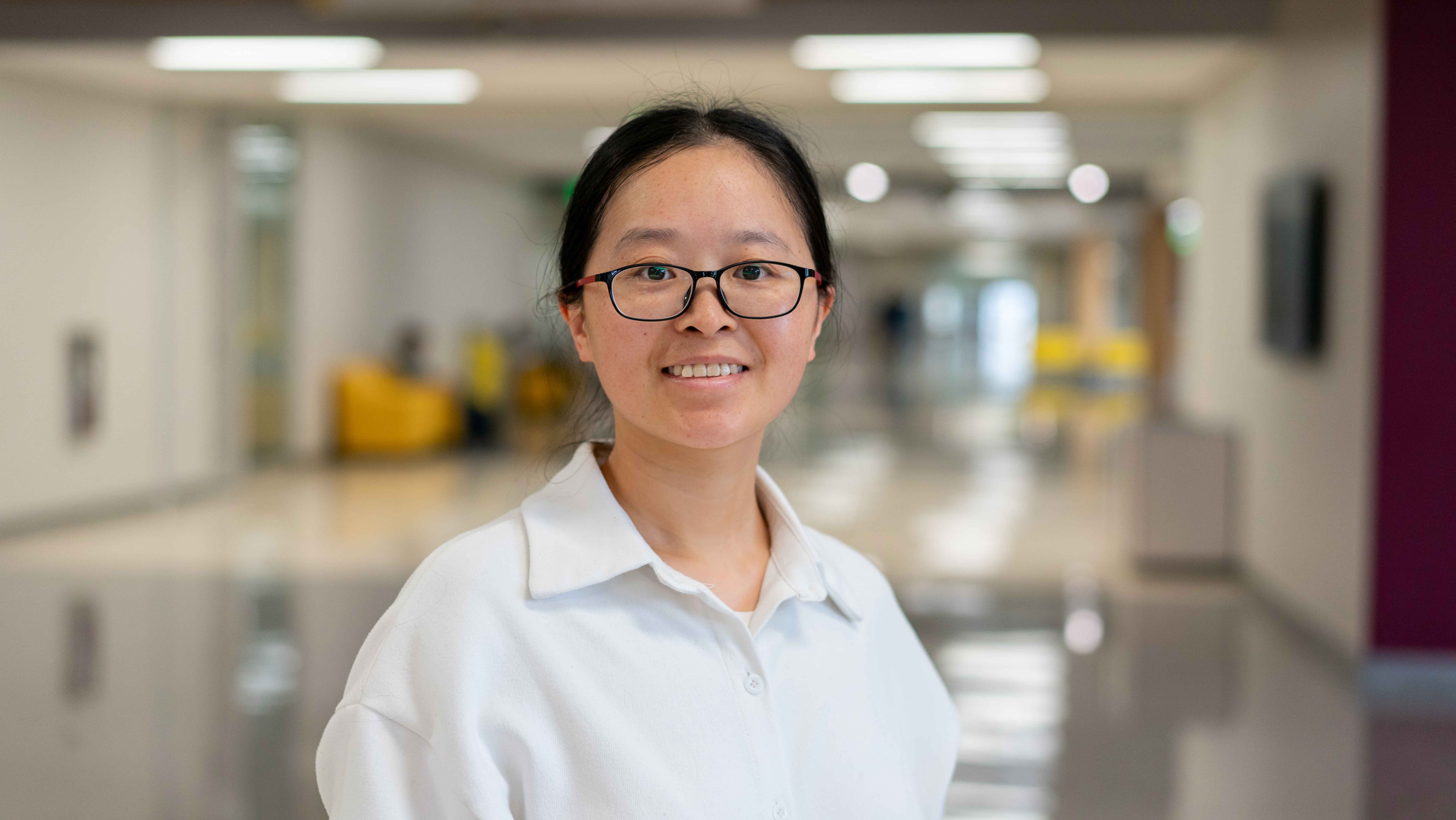
I feel recognized by this community, and this makes me feel confident and deeply honored.
As Limei Wang entered her final year as a doctoral student, she had questions. Should she pursue a career in industry or academia? If she chose academia, how should she prepare herself? In the fall of 2023, Wang participated in the Rising Stars in EECS workshop in search of answers. Launched by MIT’s Department of Electrical Engineering and Computer Science (EECS) in 2012, this annual workshop helps Ph.D. candidates and postdocs of historically underrepresented students prepare to enter the electrical engineering and computer science workforce. The workshop was hosted by the Georgia Institute of Technology.
Being selected to participate in the Rising Stars workshop was “very exciting” for Wang, a Ph.D. candidate in the Department of Computer Science and Engineering at Texas A&M University.
“To me, [EECS] is not only a workshop but a recognition,” Wang said. “It feels very different from other conferences where I’ve had a paper accepted because participants are recognized as rising stars in their areas. I feel recognized by this community, and this makes me feel confident and deeply honored.”
Wang’s Research: The First Step in Drug Discovery
Wang’s acceptance into the Rising Stars workshop is a testament to the strength of her research. Her research interests are machine learning, deep learning and graph analysis and their applications in chemistry and biology. Machine learning is a type of AI that is trained to make decisions using algorithms. Deep learning is a more intense subtype of machine learning modeled after the networks in the human brain. Deep learning can offer higher accuracy than machine learning but requires more powerful processors, larger data sets and more training time.
Wang is designing a deep learning model to make predictions about the properties and functions of molecules. She described her research as the “first step” in drug discovery — she provides a model to screen molecules with desired functions, then experts in chemistry and biology can validate the functions of top-ranking molecules through experiments.
One challenge of Wang’s research is symmetry since molecules exist in 3D space. Wang must incorporate invariant design, meaning her deep learning model will give the same prediction even if the molecule is shifted or rotated in 3D space. Models cannot make accurate predictions if they don’t understand this concept.
Dr. Shuiwang Ji, professor in the Department of Computer Science and Engineering and Wang’s faculty advisor, praised her work: “Limei has been making remarkable contributions to geometric deep learning principles and applications in solving drug discovery problems and is well-deserving of this recognition.”
At the Workshop
For Wang, there were three helpful aspects of the workshop: panel sessions, “job-talk” practice and poster presentations. The panel sessions were most important to Wang. Panelists included new professors, senior professors and one faculty member who had just moved from industry to academia. They offered advice about how to prepare for the application and interview process for faculty positions, how to excel as a junior or senior professor, and how to decide between entering industry or academia.
“The topic I was most interested in was industry versus academia,” Wang said. “[The panelists] talked about the difference and shared how they made a decision.” From these discussions and conversations with other doctoral students and postdocs at the conference, she learned that entering industry and then returning to academia is a viable option. Wang decided that this is the path she will take.
As part of the workshop, Wang also participated in mock interviews and a poster presentation where she talked about her research. The professors then offered feedback.
“The practice helped a lot,” Wang said, “especially [the advice about] how to make readers deeply interested in your topic at the beginning of your talk.”
Wang believes practicing at the workshop and the feedback she received helped her effectively introduce her research. She passed her preliminary exam shortly after the workshop and attributes her success, at least in part, to the workshop.