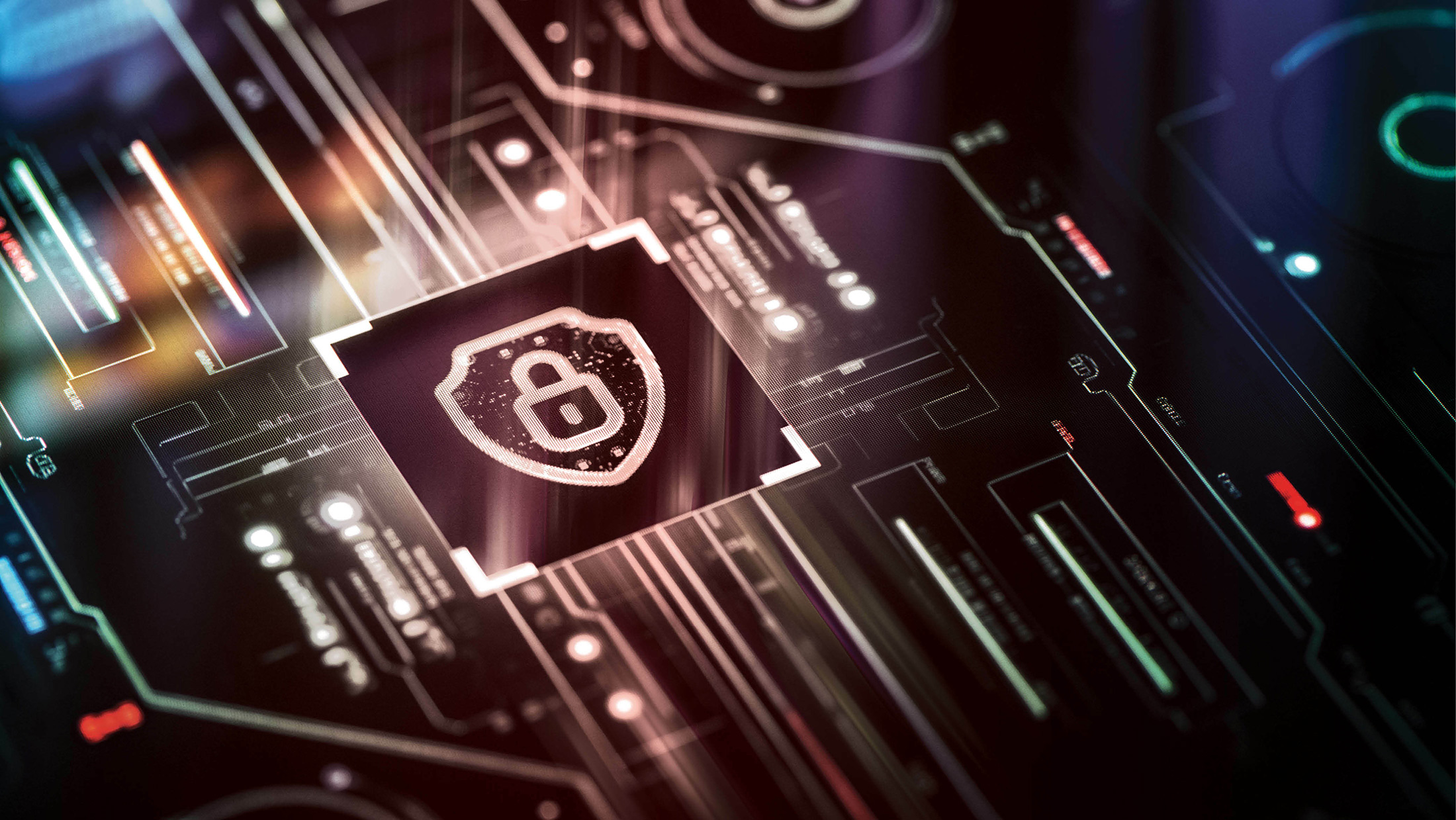
Text-generating AI programs like ChatGPT are known for everyday tasks like answering questions, but that’s not all they’re good for. These AI agents can use their text-parsing powers to help further nuclear science and assist health physicists, as shown by nuclear engineering graduate student Zavier Ndum’s research.
Large-language models (LLM) like ChatGPT are a form of artificial intelligence that uses written data to generate text based on predictions. Although LLMs are useful for many domains, such as software engineering and research assistance, their use in nuclear science is less common. This is in part because nuclear engineering research often uses proprietary data that can’t be securely fed into a general LLM.
“When it comes to nuclear science, there is a lot of proprietary data, and there are security issues with sharing data,” Ndum said. “You can't just give this knowledge to ChatGPT or Copilot. If you have something that can be used within an organization to automate workflows, it increases productivity and efficiency.”
Ndum recently wrote a paper about a project using an LLM for nuclear science. The AI agent described in Ndum’s paper, AutoFLUKA, can help automate tasks in nuclear science research, such as running computer simulations in a software called FLUKA. The application can take and edit input files, run simulations, and then analyze the results, complete with plotting graphs.
Other researchers can use Ndum’s application with their own data, securely building a database using proprietary data that doesn’t go anywhere other than the originating computer.
When it comes to nuclear science, there is a lot of proprietary data, and there are security issues with sharing data. You can't just give this knowledge to ChatGPT or Copilot.
“You're able to sort through this information very easily and very fast, instead of reading through to extract a simple answer,” Ndum said. “If you have those documents, and you have the application gain access to those documents, it can easily do Q&A, and you can prompt the model to give you suggestions to augment your specific application.”
To build AutoFLUKA as a proof of concept, Ndum had to work with what he had. He did not have access to work with Monte Carlo N-Particle (MCNP), a computer simulation code that is commonly used in nuclear science but is also regulated. However, FLUKA is similar enough to MCNP that Ndum’s model can be easily replicated for it and other simulation programs.
Another challenge for Ndum was transitioning his research focus to studying AI agents. Before arriving at Texas A&M, he worked on health physics and dosimetry, or the study of radiation absorbed by living tissue. However, after working with Dr. John Ford, a nuclear engineering professor who researches health physics, Ndum used that work to provide case studies for the AutoFLUKA paper. He also cites the guidance of nuclear engineering professor Dr. Yang Liu, his Ph.D. advisor, and Dr. Jian Tao, a College of Performance professor affiliated with the Department of Nuclear Engineering, while building the application.
“When you step into new territory, it's always challenging, but you keep on working and you believe that there is something useful you can make out of this,” he said. “When you put in that effort, it turns out good.”
Ndum, who serves as the president of the State of Texas Chapter of the Health Physics Society (STC-HPS), also hopes to bring this approach back to his original field of health physics. At the chapter’s annual conference in October at the University of Texas at Arlington, he presented a talk about the use of LLMs in health physics to serve as virtual assistants for professionals in the field. He tested the application from his paper on retrieving information from health physics-related documentation, cutting down an hours-long search to seconds. This can help health physicists like radiation safety officers (RSOs), who must know about annual radiation dose regulations and the operation of various machines.
“Sorting through these regulatory guides for RSOs could be time-consuming and strenuous,” Ndum said.
The ability to leverage AI for secure, domain-specific automation is a game-changer, and Zavier’s contributions are paving the way for more efficient, data-driven advancements in reactor modeling, health physics, and nuclear safety.
Ndum also delivered a seminar as part of a semester-long series last fall hosted by Texas A&M’s Research in Artificial Intelligence for Science and Engineering Initiative. He plans to present another talk on this topic at the STC-HPS Student Conference at Texas A&M University in April, where nuclear science, health physics, and engineering students from universities other than Texas A&M will attend.
“They can see how to harness this technology to their own specific research areas,” he said.
Currently, Ndum is advancing his research by developing a sophisticated LLM application designed to answer complex, domain-specific questions in nuclear science research. This application sorts through and analyzes documents, and it can also integrate information from online sources in real time. It can read and process multiple file types, including PDFs, images and spreadsheets, offering comprehensive support for research tasks. These abilities allow the application to provide nuanced answers and insights to make nuclear science research more efficient.
“This is new territory, and exploring it within nuclear science is really important,” Ndum said. “I'm going to keep working to see what we're able to build with this knowledge.”
Liu, who serves as the director of the Scientific Machine learning for Advanced Reactor Technologies (SMART) group, believes Zavier’s work will make an impact on the broader nuclear engineering community.
“LLMs are a research focus of our group, and we are fortunate to have Zavier pushing the boundaries of its application in nuclear science,” Liu said. “His innovative approach to integrating AI into nuclear research is exactly the kind of forward-thinking mindset we need in this field. The ability to leverage AI for secure, domain-specific automation is a game-changer, and Zavier’s contributions are paving the way for more efficient, data-driven advancements in reactor modeling, health physics, and nuclear safety.”